When you apply, we will ask you to provide a ranking of prospective mentors and select from a list of research projects that you would potentially be interested in working on. To frame the social relevance of the research, each project is aligned with at least one Sustainable Development Goal (SDG) defined by the United Nations.
Your mentor rankings and project selections are important because they will determine which people will evaluate your application. We therefore encourage applicants to explore each mentor’s website to learn more about the individual research activities of each lab.
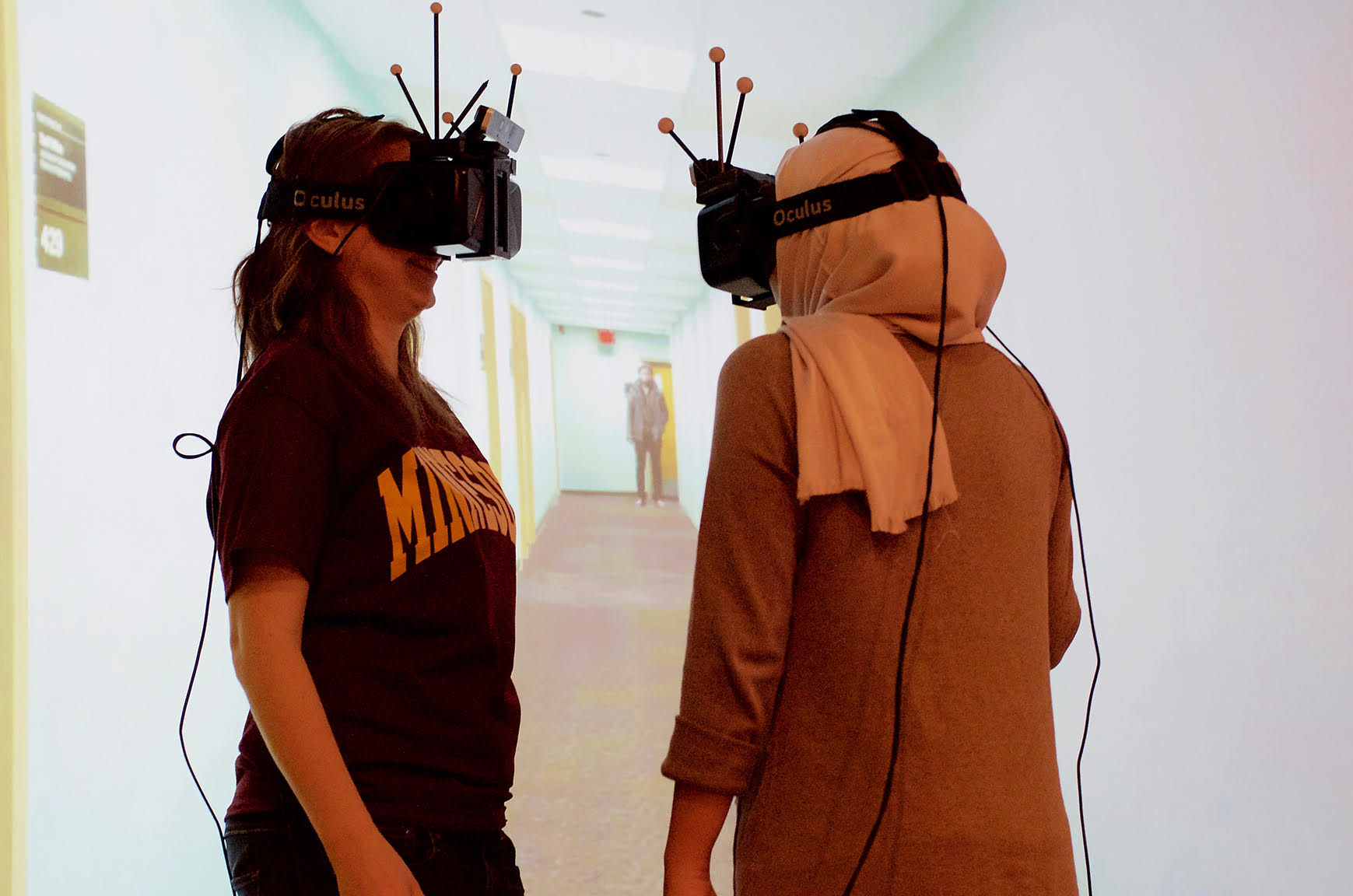
SDG: Good Health and Well-Being
International research has shown multiple benefits to health and well-being from exposure to natural environments ("forest-bathing"). In some situations, such as for populations who have limited access to real-life nature immersion experiences, such as elderly residents of care homes, or for people who are temporarily undergoing a stressful experience from which respite would be welcome, such as chemotherapy or dialysis patients, virtual reality-based nature immersion may offer some value. In this project, the summer intern will work with an interdisciplinary team of researchers to explore fundamental questions informing the optimal deployment of VR technologies for this purpose.
Mentor: Zhu-Tian Chen
SDGs: Decent Work and Economic Growth, Sustainable Cities and Communities
Augmented Reality (AR) has shown potential to assist individuals across a wide spectrum of disabilities, including those with visual impairments, hearing difficulties, and cognitive disorders, by leveraging its real-time, multi-modal capabilities. This project seeks to harness the power of AR through an intelligent agent designed to assist individuals in their daily tasks. Our AR agent will serve as an assistive tool to foster an inclusive environment for individuals in various contexts, including workplaces, educational settings, and public areas, making our daily environments safer, more intelligent, and more welcoming for everyone, especially those in vulnerable situations.
Explaining Medical Decisions Made by AI
Mentor: Qianwen Wang
SDG: Good Health and Well-Being
AI is being progressively employed in medical domains, including medical image diagnostics and personalized medicine. While these applications offer significant advantages, especially in regions and among patients with limited medical resources, they also bring forth challenges such as potential biases in decisions (e.g., disparities in accuracy based on race and gender) and a lack of transparency (e.g., patients do not know why a certain decision is made and whether they should trust it). The objective of this project is to create interactive visualization tools that empower users to comprehend the decisions made by medical AI models, detect potential biases, and make informed decisions for their health and well-being. In this project, students will explore different explainable AI algorithms and combine them with interactive visualization techniques to decode AI decision-making processes, breaking them down into simpler and understandable components.
Designing and Evaluating Human-Centered Explainable AI
Mentor: Harmanpreet Kaur
SDG: Reduced Inequalities
AI- and ML-based systems are now routinely deployed in the wild, including in sensitive domains like criminal justice, public policy, healthcare, and education. Harmful use of AI/ML can only be avoided if the people who build and use these systems understand the reasoning behind their predictions. Researchers and practitioners have developed approaches like interpretability and explainability to help people understand model outputs and reasoning. This project will build on findings from prior work to: (1) continue evaluating interpretable ML and explainable AI techniques on whether they meet the criteria of human understanding and cognition, and (2) design ways to actively support human understanding of complex AI and ML outputs. To do so, we will translate relevant work from cognitive, social, and learning sciences for this AI/ML context. Students will work on the full AI/ML pipeline, including data gathering and preprocessing, data modeling, and understanding data using existing interpretability and explainability tools, and learn how to conduct human subjects research on this topic.
Computational Support for Recovery from Addiction
Mentor: Lana Yarosh
SDG: Good Health and Well-Being
Addiction and alcoholism are one of the greatest threats to people’s health and well-being. Early recovery is a particularly sensitive time, with as many as three-fourths of people experiencing relapse. Computing provides an opportunity to connect people with the support they need to get and stay in recovery. Working closely with members of the recovery community, we will conduct and analyze qualitative formative work to understand people's needs and values. Based on insights gained from this work, students will contribute to the development and deployment of interactive technologies for computational support for recovery. As some examples, we may work on a conversational agent to help connect people with support or web-based writing support tools to help people write more helpful comments in online health communities.
Immersive Data Storytelling for Climate Action
Mentor: Daniel Keefe
SDG: Climate Action
This project explores new methods for bringing high-end 3D scientific data visualization to a wider audience. We will target two delivery platforms: 3D web (for the widest possible dissemination) and science museum planetarium domes (for a compelling immersive display experience). The project will leverage a long-term existing lab partnership with the Bell Museum of Natural History, which is located on campus and accessible via campus transportation. The project is a perfect fit for computer science students who also have an interest in writing, storytelling, filmmaking, and/or visual art. The research will make use of a custom 3D data visualization engine developed via the NSF-funded Sculpting Visualizations project (an art-science collaboration) and reimagine the computer graphics software as an interactive data storytelling platform. Also drawing on existing collaborations, we will work directly with climate scientists and data from their latest supercomputer simulations to, for example, tell the story of what is happening to the Ronne Ice Shelf in Antarctica.
Towards Safer Robotic Colorectal Surgeries: Visual Scene Recognition in the Abdominal Cavity to Prevent Inadvertent Surgical Injuries
Mentor: Junaed Sattar
SDG: Good Health and Well-Being
This project will focus on developing automated strategies for surgical robots to understand the visual images seeing through a laparoscopic camera during colorectal surgery. The end goal is to ensure the prevention of surgical injuries and minimize post-surgical recovery cost and time, and improve patient well-being. The project will build upon the work of the interactive robotics and vision laboratory, particularly in the space of visual scene classification and panoptic segmentation in real-time, and deep-learned saliency-based attention modeling for human-robot interaction for surgical tasks.
Finding Trash: Algorithmic Search Strategies for Locating Debris with Autonomous Underwater Robots
Mentor: Junaed Sattar
SDGs: Life Below Water; Clean Water and Sanitation
This project will focus on developing search strategies for autonomous underwater robots to find trash and debris underwater, with the long-term goal of removing such debris to conserve the health of the aquatic and marine ecosystems. The project will build upon the work of the interactive robotics and vision laboratory, in which underwater robots equipped with imaging sensors have been used to detect and localize crashes underwater in cooperation with human divers. The majority of underwater trash, which is also a significant health hazard, comprises plastic materials known to deform easily. This project will combine the trash detection capabilities of robots with algorithmic search strategies to efficiently find, and eventually remove these items, which pose a significant threat to the health of marine flora and fauna.
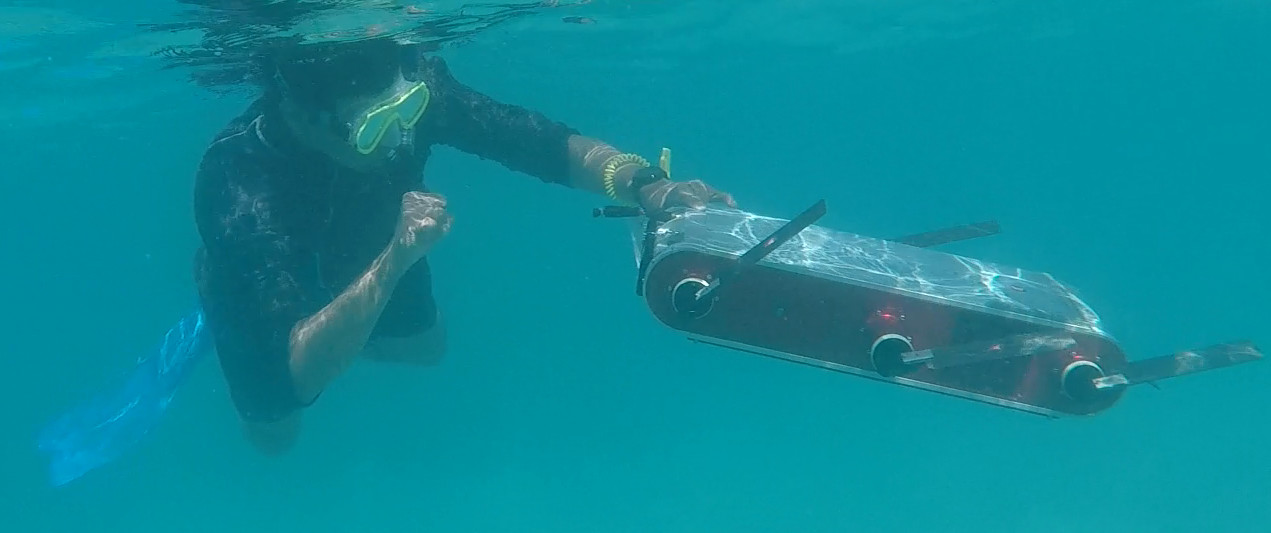